Summary
Our crop physiology program focuses on understanding ways in which plant physiological processes are integrated to cause whole plant responses under field conditions and how these processes can be manipulated to improve the management of crops. It focus in understanding the dynamics of the mass and energy balance of crops. More specifically, optimizing the balance between canopy light capture and biomass accumulation (photosynthesis) and water losses through the evapotranspiration process under the dryland crop production systems of the lower coastal bend region of Texas.
Phenotyping for Dryland Production
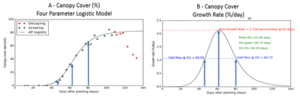
Example of time course of canopy cover development collected using Unmanned Aerial System (A) and growth rate (B) for Phytogen 499 WRF derived from UASdata atCorpus Christi, TX, 2016.
Phenotyping is a term used in plant breeding to describe characteristics or traits of a genotype. The objectives of this project are to demonstrate the potential of using unmanned Aircraft System (UAS) platforms for high-throughput phenotyping for genotype evaluation and selection purposes. Plant growth and growth rate response curves displayed in figure 1 enabled the extraction of additional growth and rate parameters to characterize the performance of each genotypes studied. Thirteen parameters can be extracted for each trait (plant height, canopy cover, and canopy volume). The data analysis procedure demonstrates the feasibility and capability of using UAS-based platforms, combined with data processing software, to extract plant growth data capable of describing the temporal development and yield response of a crop. Information generated by this UAS system should be valuable to breeders in selecting or designing genotypes for a given environment. It can be beneficial to cotton agronomists, consultants, and producers in selecting cultivars with desirable traits for a production system. Our goal is to use the large volume of plant growth data generated by this platform to develop “expert systems” to automatically sort and analyze the information, identifying the most promising genotypes for a given location or production system.
Conservation Tillage and Crop Rotation Effects on Long Term Yield
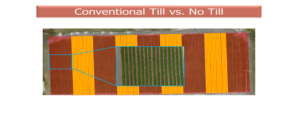
Field plot layout. Red = Conventional till (incorporating previous crop residues), Yellow=conservation till (leaving crop residues on surface).
This study presents a novel approach to use multi-temporal data for comparison of two management practices in cotton/sorghum rotation, conventional tillage (CT) and no-tillage (NT) (Fig. 2). The plant parameters considered for the comparison are canopy height (CH), canopy cover (CC), canopy volume (CV), and Normalized Difference Vegetation Index (NDVI). The test plots were divided into approximately one square meter size grids. Measurements were extracted grid wise using high resolution remote sensing data captured ten times over whole crop growing season of the cotton plots. One tailed Z-test hypothesis reveals that there is a significant difference between cotton growth under CT and NT for almost all the epochs. With 95% confidence interval, the crop grown under NT found to have taller canopy, higher canopy cover, bigger biomass, and higher NDVI, as compared to those under CT cropping system.
Develop a procedure to quantify cotton crop maturity index
Maturity index of a cultivar is an important parameter for the management of cotton crops as it affects planting date, seeding rate, timing and rate of growth regulator applications, last irrigation and nutrient application, and finally the rate and timing of harvest aid applications. Field plots will be established at Corpus Christi, Texas, under Irrigated and dryland conditions to develop a physiology-based maturity index for 25 commercial cultivars with a wide range of maturities, as determined by seed providers. Selected cultivars will represent the following maturity groups; early, early-mid, medium, medium-late, and late maturity. The plots (4 rows, 10 m long) will be planted with four replications in a split plot design with water regime as main plots and cultivars as sub-plots. UAS data will be collected weekly from emergence to harvest using RGB and multi-spectral sensors. Images from these flights will be used to extract temporal plant growth parameters. Temporal plant growth parameters, vegetative indexes (NDVI, ExG), bloom rate, and boll opening estimates will be used to rank the maturity pattern of each cultivar. These rankings will be correlated with traditional maturity parameters such as nodes above white flower, nodes above last cracked boll, and time to 60 % open boll.
Mepiquat Chloride: Prescription Application Rate Model
Mepiquat Chloride (MC) is a plant growth regulator, commonly used in commercial cotton production to control excessive vegetative growth. MC alters the concentration of Gibberellic Acid (G.A.) in cells by inhibiting the synthesis of G.A. in the plant. Field plots will be established at Corpus Christi, Texas, under dryland conditions. The plots will include nine commercial cultivars with differences in maturity (early, medium, and late) from commercial seed companies. MC treatment will be (1) untreated, and (2) rates applied to each entry based on a model developed using UAS collected parameters (plant height and canopy cover). The plots (4 rows, 10 m long) will be planted in a split plot design, with MC application methods as main plots and cultivars as sub-plots. UAS data will be collected weekly from emergence until harvest using RGB and MULTISPECTRAL sensors. Temporal plant growth parameters in terms of plant height, canopy cover, and canopy volume will be used to develop a model based on plant volume/weight estimates. This plant biomass estimates will be used to develop a relationship to determine the rate of MC needed to reach a desire MC concentration in the plant, following the procedure developed by Landivar in previous studies. The effectiveness of the treatments will be evaluated in terms of changes in plant height and lint yield.
Harvest-aid model to estimate the time and rate of application
Senescence is the final stage of leaf development and crop maturity. The application of harvest-aid chemicals is a widely accepted cultural practice to induce leaf abscission of cotton foliage to facilitate mechanical harvest. These compounds interact with environmental and physiological factors to promote leaf drop and allow timely and efficient harvest of the lint to reduce harvest losses from weathering. Field plots will be established at Corpus Christi, Texas, under dryland conditions. The plots will include nine commercial cultivars with differences in maturity (early, medium, and late) from commercial seed companies. Harvest aid treatments will be (1) single application based on the maturity estimates for the check cultivar (a mid-season cultivar) and (2) rates applied to each entry based on a model developed using UAS collected parameters. The plots (4 rows, 10 m long) will be planted in a split plot design, with harvest-aid application methods as main plots and cultivars as sub-plots. UAS data will be collected weekly from emergence until harvest using RGB and MULTISPECTRAL sensors. Temporal plant growth parameters and vegetative indexes (NDVI and ExG) will be used to develop crop maturity estimates. Plant growth rates will be used to develop a parameter to indicate the time of harvest-aid applications. NDVI and/or ExG data will be used to determine the rate of application. The accuracy of the UAS forecasted time of application will be validated with field collected data on time for 60% boll opening and nodes above the uppermost cracked boll.
Project Leader
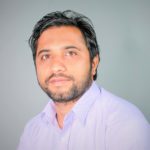
Dr. Mahendra Bhandari
Team Members
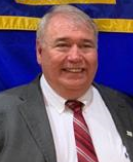
Dr. Juan Landivar-Bowles
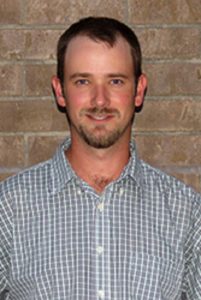
Dr. Joshua McGinty
Publications
-
- J. Jung, M. Maeda, A. Chang, M. Bhandari, A. Ashapure, J. Landivar, “The potential of remote sensing and artificial intelligence as tools to improve the resilience of agriculture production systems”, Current Opinion in Biotechnology, vol. 70, pp. 15-22, 2020.
- A. Ashapure, J. Jung, A. Chang, S. Oh, J. Yeom, M. Maeda, A. Maeda, N. Dube, J. Landivar, S. Hague, W. Smith, “Developing a machine learning based cotton yield estimation framework using multi-temporal UAS data”, ISPRS Journal of Photogrammetry and Remote Sensing, vol. 169, pp. 180-194, 2020.
- S. Oh, A. Chang, A. Ashapure, J. Jung, N. Dube, M. Maeda, D. Gonzalez, J. Landivar, “Plant Counting of Cotton from UAS Imagery Using Deep Learning-Based Object Detection Framework”, Remote Sensing, 12(18):2981, DOI: 10.3390/rs12182981, 2020.
- M. Bhandari, A. Ibrahim, Q. Xue, J. Jung, A. Chang, J. Rudd, M. Maeda, N. Rajan, H. Neely, J. Landivar, “Assessing winter wheat foliage disease severity using aerial imagery acquired from small Unmanned Aerial Vehicle (UAV)”, Computers and Electronics in Agriculture, 176:105665, DOI: 10.1016/j.compag.2020.105665, 2020.
- A. Chang, J. Jung, M. Maeda, J. Landivar, H. Carvalho, J. Yeom, “Measurement of Cotton Canopy Temperature Using Radiometric Thermal Sensor Mounted on the Unmanned Aerial Vehicle (UAV)”, Journal of Sensors, 2020:1-7, DOI: 10.1155/2020/8899325, 2020.
- A. Ashapure, J. Jung, A. Chang, S. Oh, M. Maeda, J. Landivar, “A comparative study of RGB and multispectral sensor based cotton canopy cover modelling using multi-temporal UAS Data,” Remote Sensing, 11(23):2757, DOI: 10.3390/rs11232757, 2019.
- J. Yeom, J. Jung, A. Chang, A. Ashapure, M. Maeda, A. Maeda, J. Landivar, “Comparison of Vegetation Indices Derived from UAV Data for Tillage Treatment in Agriculture,” Remote Sensing, 11(13):1548, DOI: 10.3390/rs11131548, 2019.
- A. Ashapure, J. Jung, J. Yeom, A. Chang, M. Maeda, A. Maeda, J. Landivar, “A novel framework to detect conventional tillage and No-tillage cropping system effect on cotton growth and development using multi-temporal UAS data,” ISPRS Journal of Photogrammetry and Remote Sensing, 152, pp. 49-64, 2019.
- J. Enciso, C.A. Avila, J. Jung, S. Elsayed-Farag, A. Chang, J. Yeom, J. Landivar, M. Maeda, J.C. Chavez, “Validation of agronomic UAV and field measurements for tomato varieties,” Computers and Electronics in Agriculture, 158, pp. 278-283, 2019.
- Yang, Y., L.T. Wilson, J. Jifon, A. Landivar, J. da Silva, M.M. Maeda, J. Wang, E. Christensen, 2018. Energy cane growth dynamics and potential early harvest penalties along the Texas Gulf Coast. Biomass and Bioenergy 113, 1-14.
- J. Yeom, J. Jung, A. Chang, M. Maeda, J. Landivar, “Automated Open Cotton Boll Detection for Yield Estimation Using Unmanned Aircraft Vehicle (UAV) Data,” Remote Sensing, doi:10.3390/rs10121895, 2018.
- J. Jung, M. Maeda, A. Chang, J. Landivar, J. Yeom, J. McGinty, “Unmanned Aerial System assisted framework for the selection of high yielding cotton genotypes,” Computers and Electronics in Agriculture, 152, pp. 74-82, 2018.
- J. Enciso, M. Maeda, J. Landivar, J. Jung, A. Chang, “A ground based platform for high throughput phenotyping”, Computers and Electronics in Agriculture, 141, pp. 286-291, 2017.
- Chen, R., T. Chu, J.A. Landivar, C. Yang and M.M. Maeda. 2017. Monitoring cotton (Gossypium hirsutum L.) germination using ultrahigh-resolution UAS images. Precis Agric: 1-17.
- A. Chang, J. Jung, M. Maeda, J. Landivar, “Crop height monitoring with digital imagery from Unmanned Aerial System (UAS),” Computers and Electronics in Agriculture, 141, pp. 232-237, 2017.
- J. Goolsby, J. Jung, J. Landivar, W. McCutcheon, R. Lacewell, R. Duhaime, D. Baca, R. Puhger, H. Hasel, K. Varner, B. Miller, A. Schwartz, A. Perez de Leon, “Evaluation of Unmanned Aerial Vehicles (UAVs) for detection of cattle in the Cattle Fever Tick Permanent Quarantine Zone,” Subtropical Agriculture and Environments, 67, pp. 24-27, 2016.
- Tianxing Chu, Ruizhi Chen, Juan A. Landivar, Murilo Maeda, Chenghai Yang, Michael Starek. 2016. Cotton growth modeling and assessment using unmanned aircraft system visual-band imagery. Journal of Applied Remote Sensing. 10(3). http://dx.doi.org/10.1117/1.JRS.10.036018